PIONEER includes de-identified/pseudonymised data from patients who were seen by an acute care provider from 1st January 2000 and will include data from patients until the project closes (2025 at the earliest). Each dataset will be bespoke, creating to match the specific project.
Some of our datasets are listed below, but each can be finessed or expanded to meet your direct requirements, and many other conditions, pathways or therapy areas are available. If you don’t see what you need, please contact a member of the PIONEER team.
Specialist datasets available
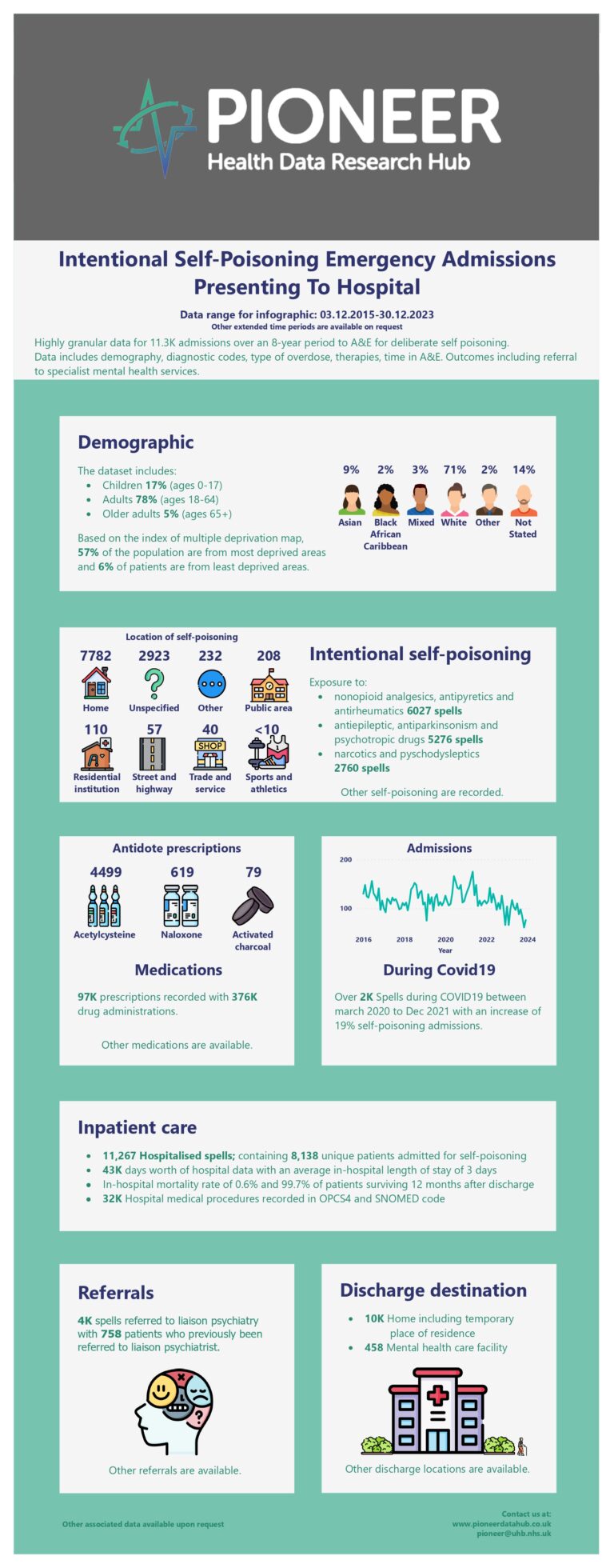
Intentional Self-Poisoning Emergency Admissions Presenting to Hospital
Highly granular data for 11.3k admissions over an 8-year period to A&E for deliberate self-poisoning. Data includes demography, diagnostic codes, type of overdose, therapies, time in A&E. Outcomes including referral to specialist mental health services.
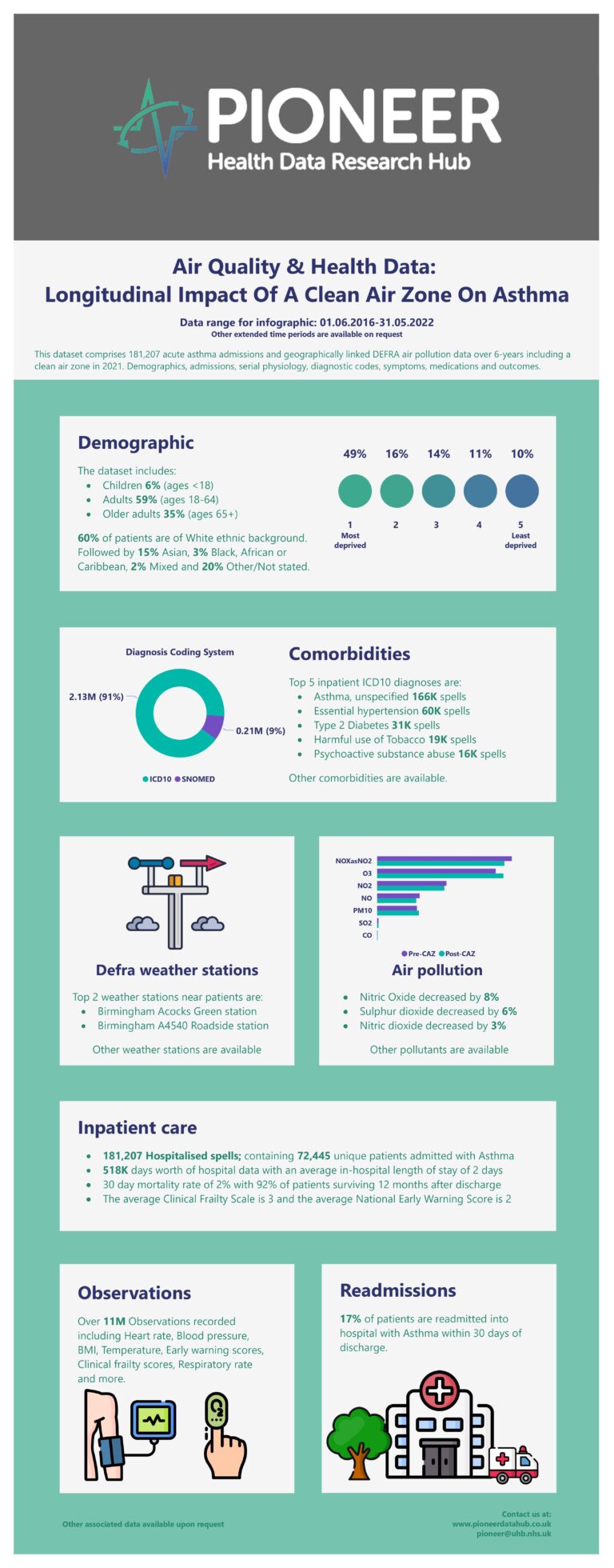
Air Quality & Health Data: Longitudinal Impact of a Clean Air Zone on Asthma
This dataset comprises 181,207 acute asthma admissions and geographically linked DEFRA air pollution data over 6-years including a clean air zone in 2021. Demographics, admissions, serial physiology, diagnostic codes, symptoms, medications and outcomes.
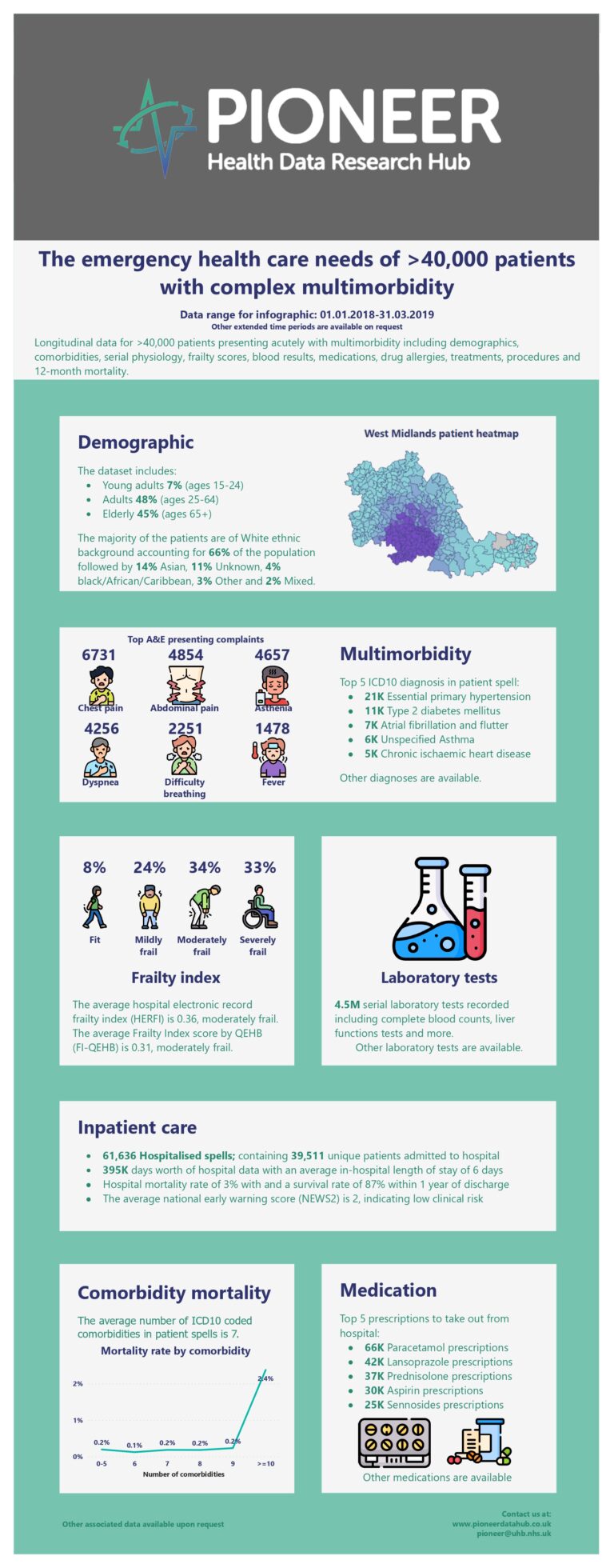
The Emergency Health Care Needs of >40,000 Patients with Complex Multimorbidity
Longitudinal data for >40,000 patients presenting acutely with multimorbidity including demographics, comorbidities, serial physiology, frailty scores, blood results, medications, drug allergies, treatments, procedures and 12-month mortality.
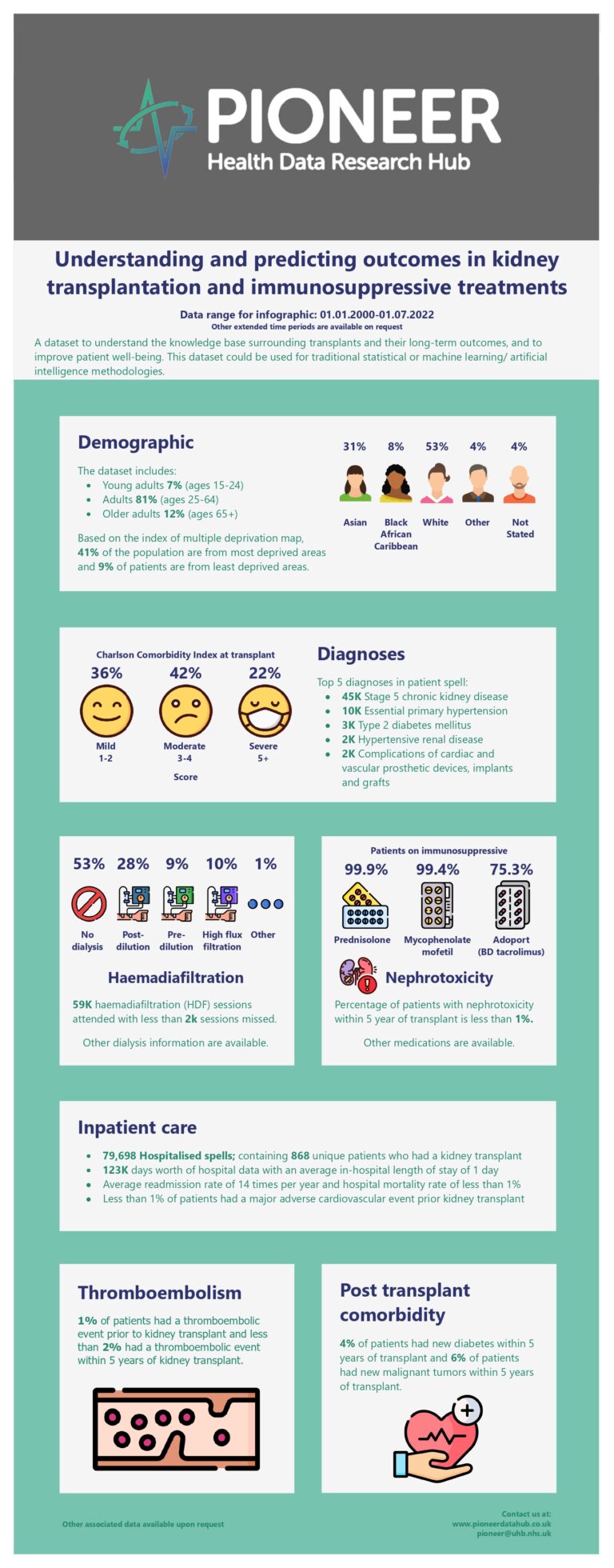
Transplants in Renal Disease: Outcomes and the Effects of Immunosuppression
Detailed dataset of >860 patients following a renal transplant on immunosuppressive treatments curated by PIONEER (Jan 2000 to Jul 2024). Granular pathways. Deeply phenotyped. Serial physiology, blood markers, demography, outpatient data and outcomes.
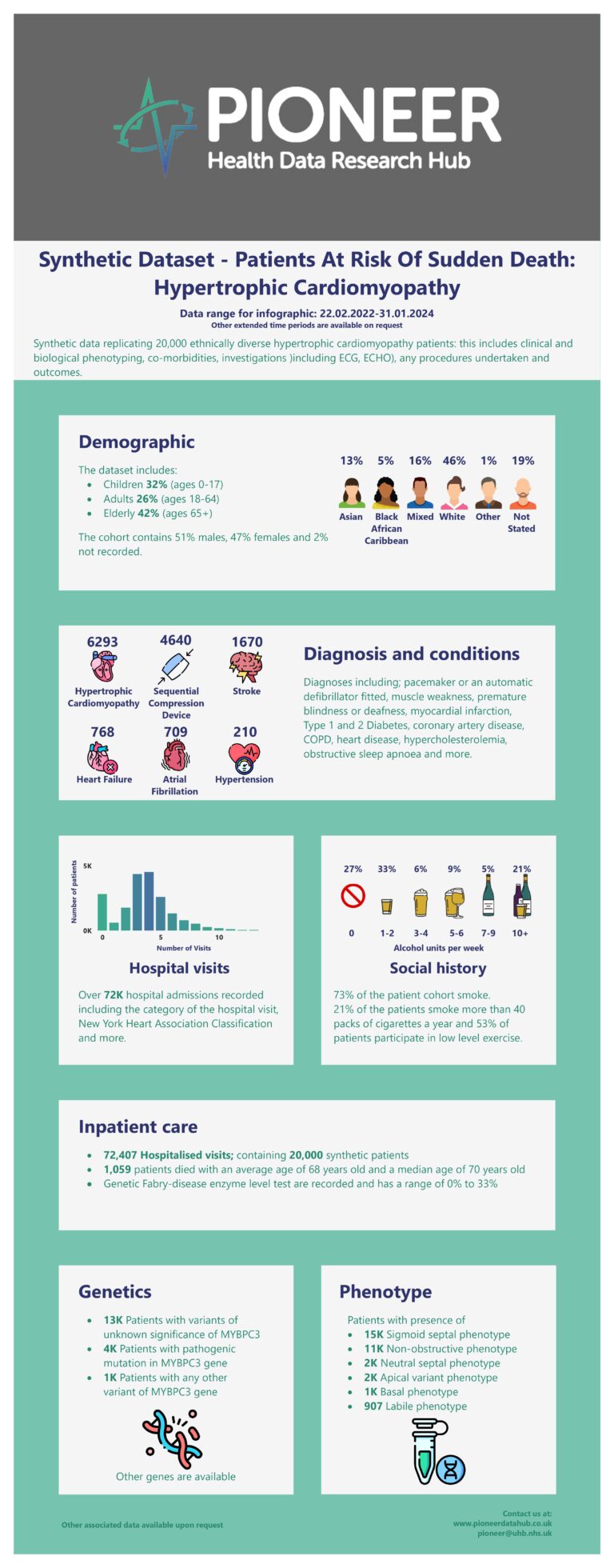
Synthetic Dataset – Patients at Risk of Sudden Death: Hypertrophic Cardiomyopathy
Synthetic data replicating 20,000 ethnically diverse hypertrophic cardiomyopathy patients: This includes clinical and biological phenotyping, co-morbidities, investigations (including ECG, ECHO), any procedures undertaken and outcomes.
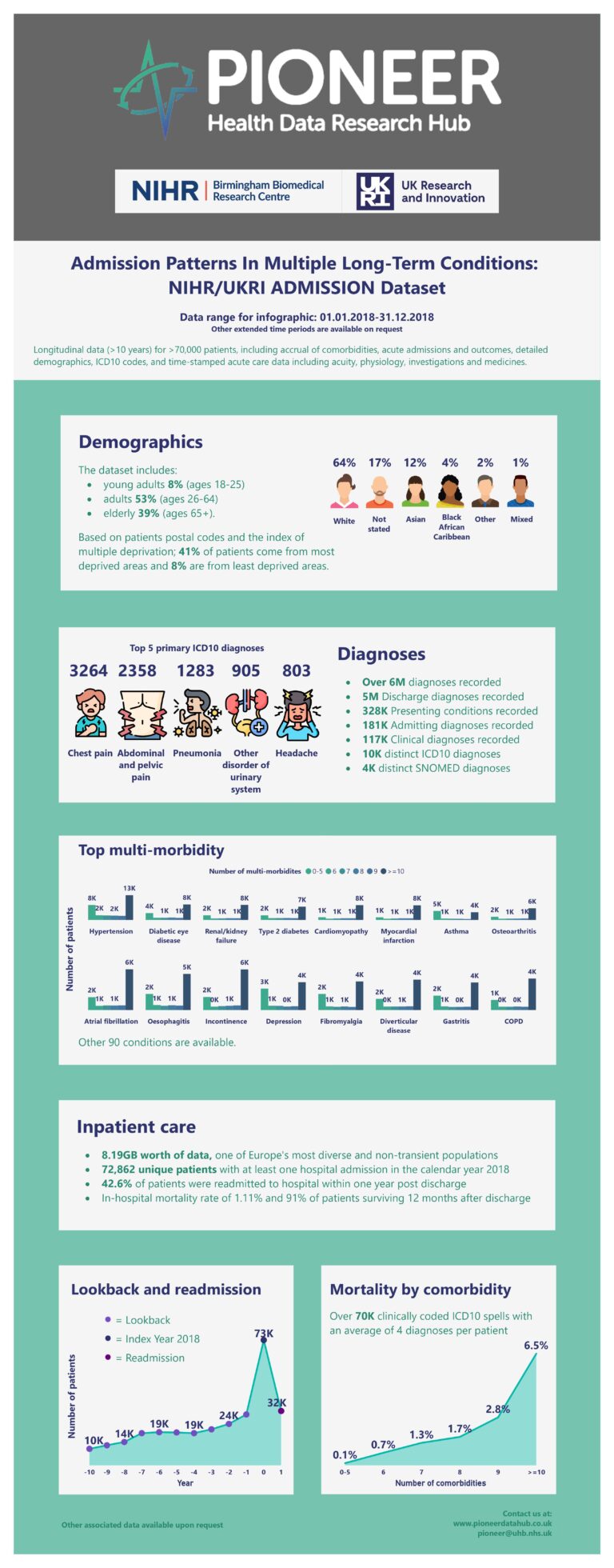
Admission Patterns in Multiple Long-Term Conditions: NIHR/UKRI ADMISSION Dataset
Longitudinal data (>10-years) for >70,000 patients, including accrual of comorbidities, acute admissions and outcomes, detailed demographics, ICD-10 codes, and time-stamped acute care data including acuity, physiology, investigations, and medicines.
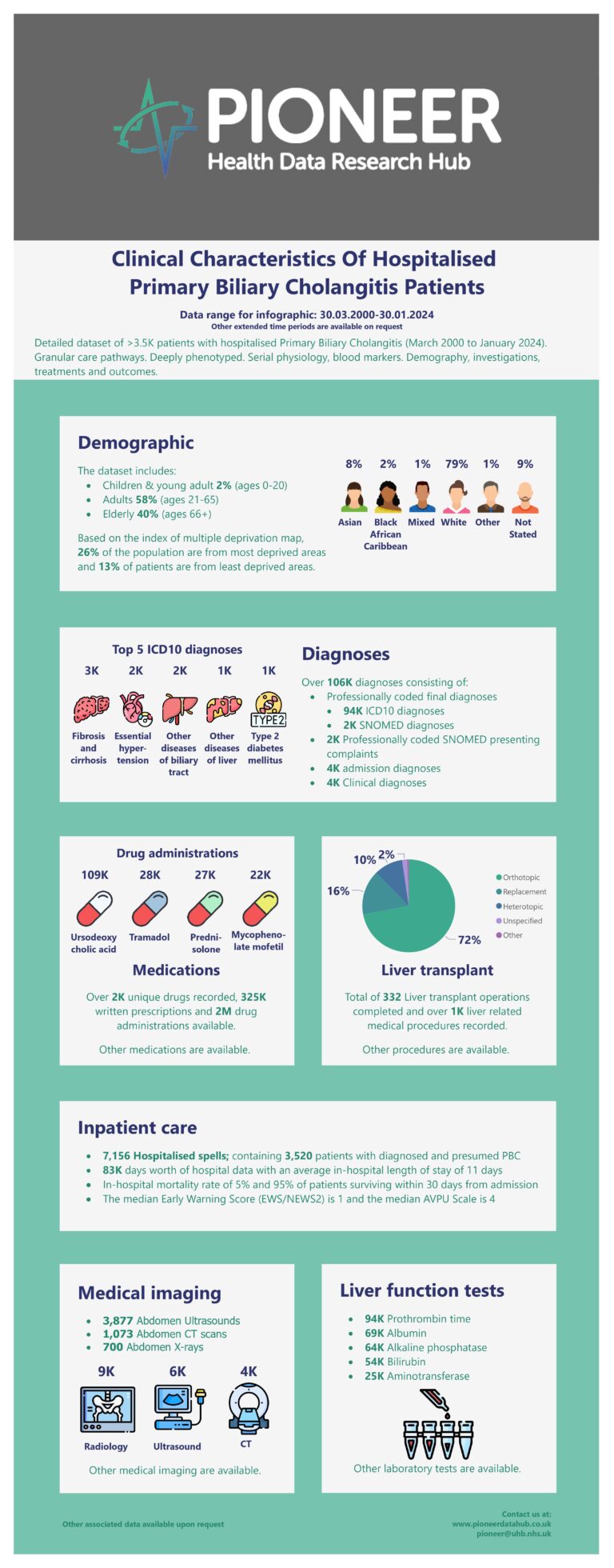
Clinical Characteristics of Hospitalised Primary Biliary Cholangitis Patients
Detailed dataset of >3.5k patients with hospitalised Primary Biliary Cholangitis (March 2000 to January 2024). Granular care pathways. Deeply phenotyped. Serial physiology, blood markers. Demography, investigations, treatments and outcomes.
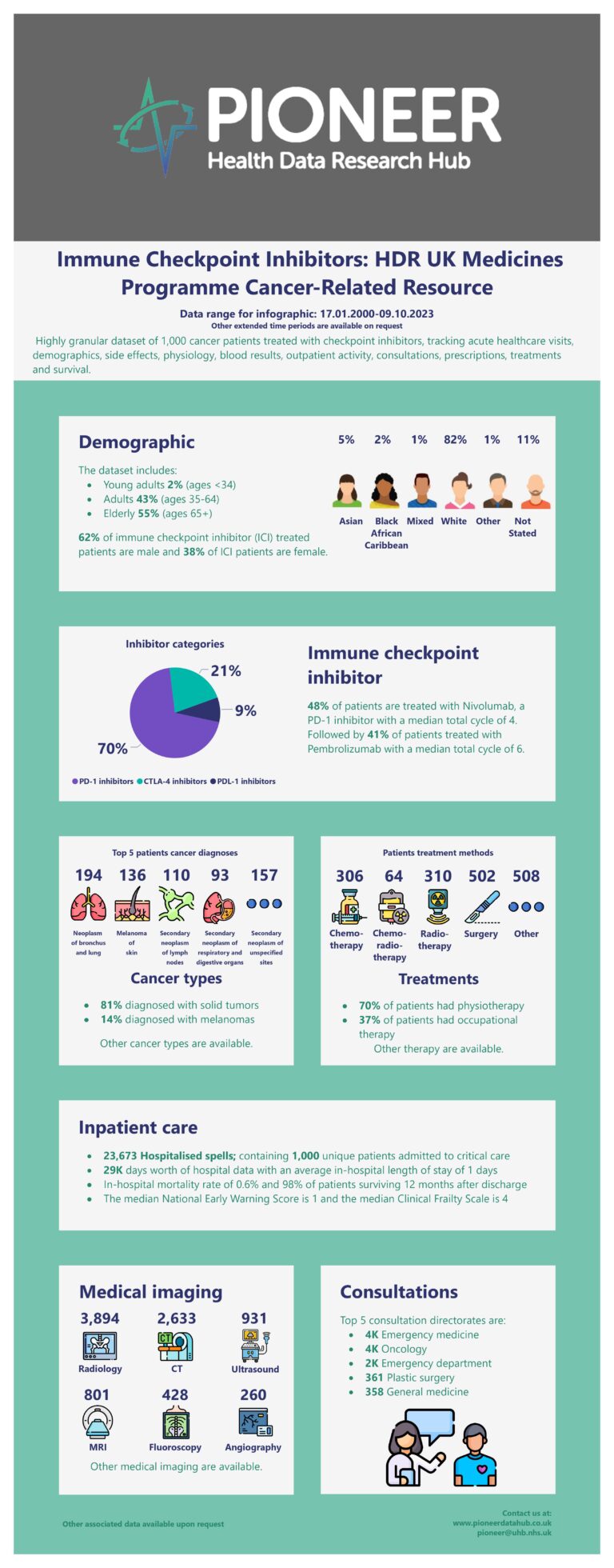
Immune Checkpoint Inhibitors: HDR UK Medicines Programme Cancer-Related Resource
Highly granular dataset of 1,000 cancer patients treated with checkpoint inhibitors, tracking acute healthcare visits, demographics, side effects, physiology, blood results, outpatient activity, consultations, prescriptions, treatments, and survival.
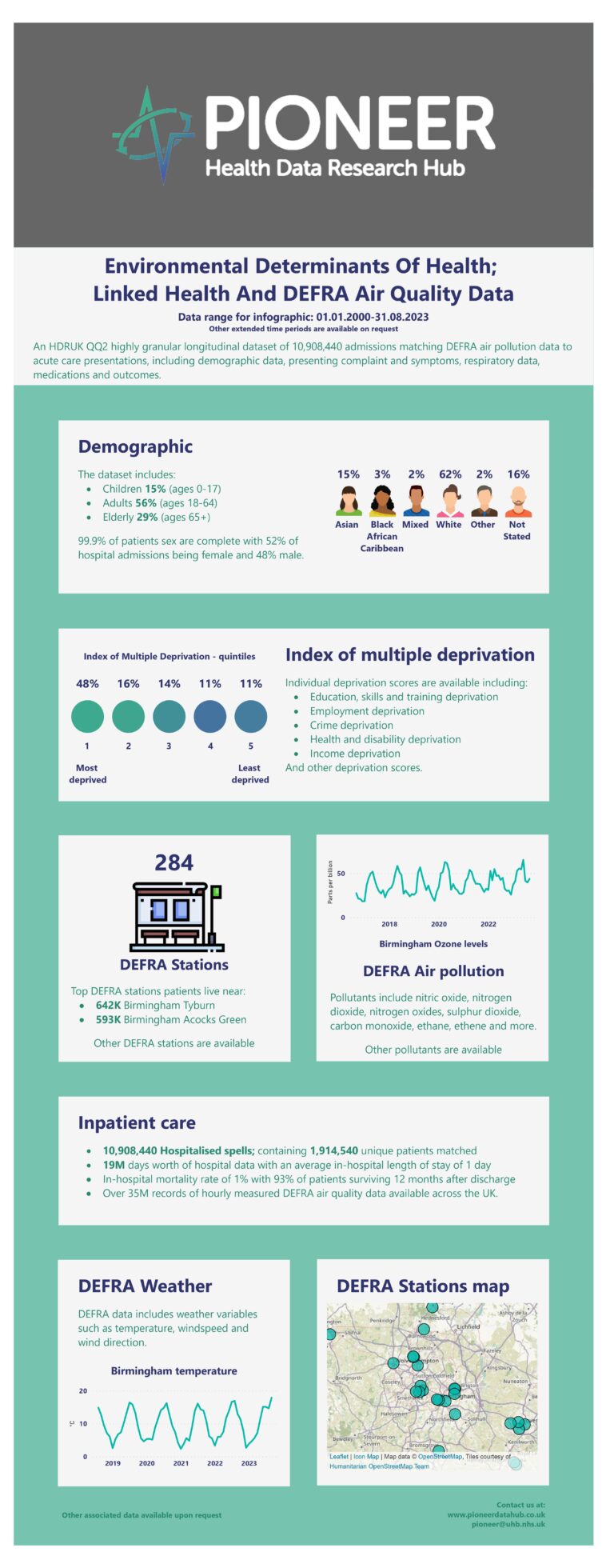
Environmental Determinants of Health: Linked Health and DEFRA Air Quality Data
An HDRUK QQ2 highly granular longitudinal dataset of 10,908,440 admissions matching DEFRA air pollution data to acute care presentations, including demographic data, acuity, presenting complaint and symptoms, respiratory data, medications and outcomes.
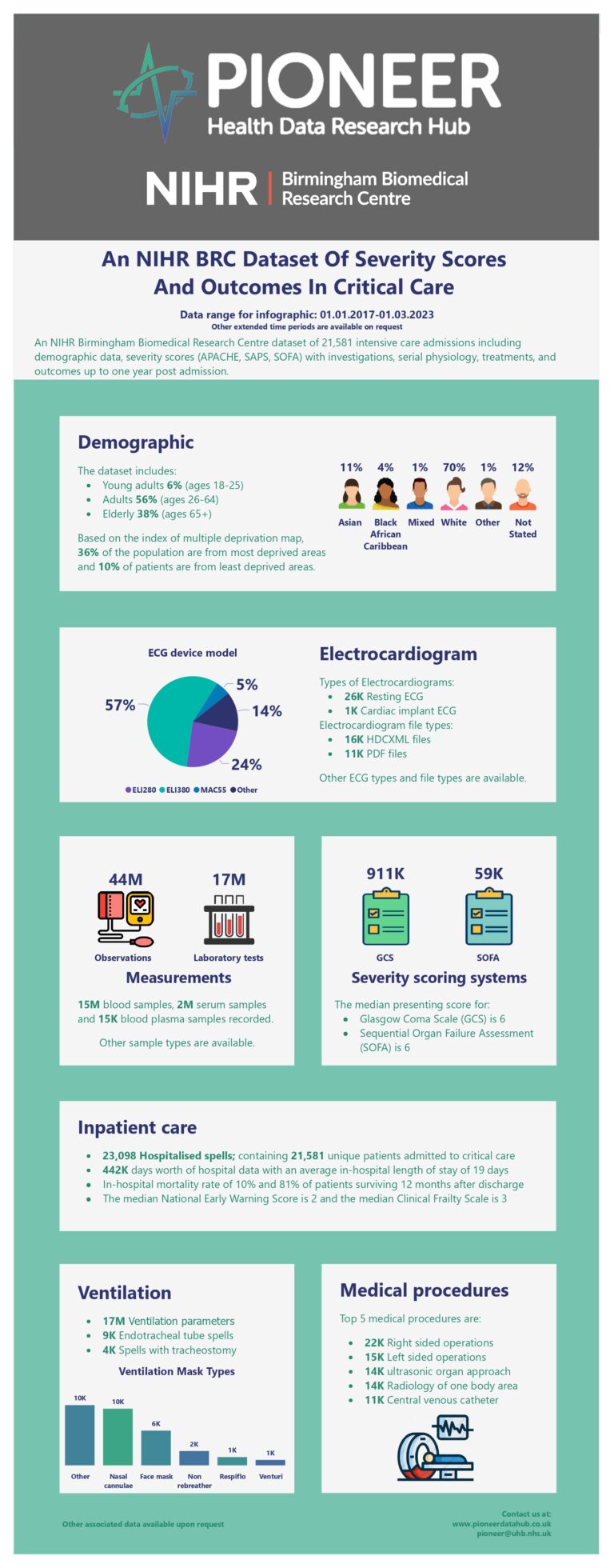
An NIHR Birmingham BRC Dataset of Severity Scores and Outcomes in Critical Care
An NIHR Birmingham Biomedical Research Centre dataset of 21,581 intensive care admissions including demographic data, severity scores (APACHE, SAPS, SOFA) with investigations, serial physiology, treatments, and outcomes up to one year post admission.
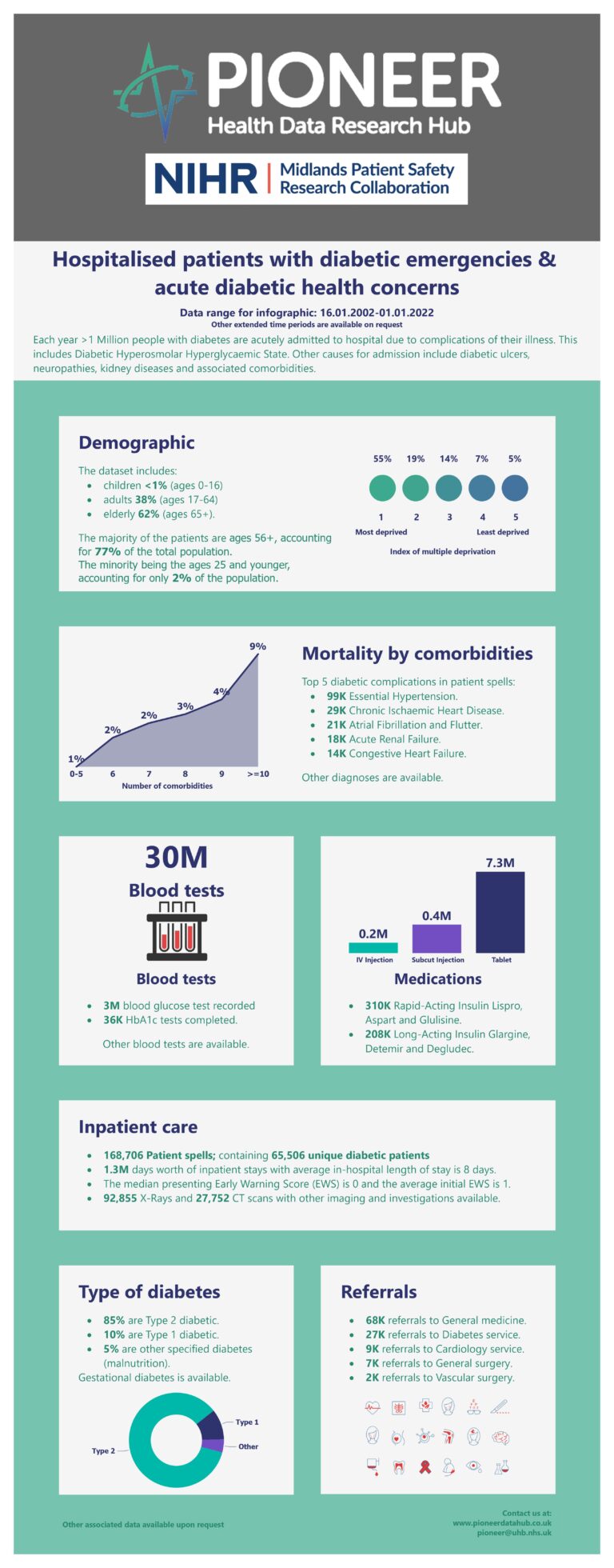
Hospitalised Patients with Diabetic Emergencies & Acute Diabetic Health Concerns
An NIHR Midlands Patient Safety Research Centre dataset of 168,706 diabetic emergencies and acute admissions associated with diabetes-related health concerns, including demographic data with investigations, serial physiology and outcomes.
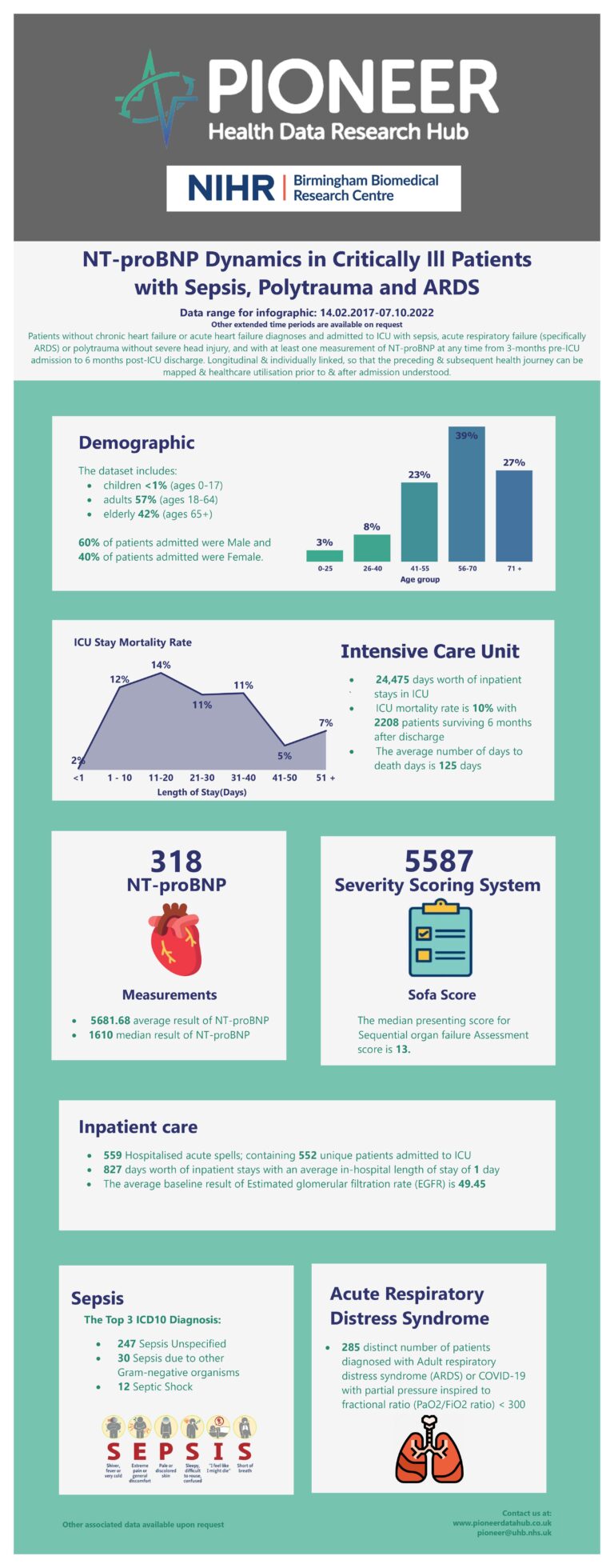
NT-proBNP in Critically Ill Patients with Sepsis: a NIHR Birmingham BRC Dataset
A National Institute for Health and Care Research (NIHR) Birmingham Biomedical Research Centre (BRC) dataset featuring serial NT-proBNP measurements from a diverse cohort of Intensive Care patients with sepsis, ARDS, and polytrauma.
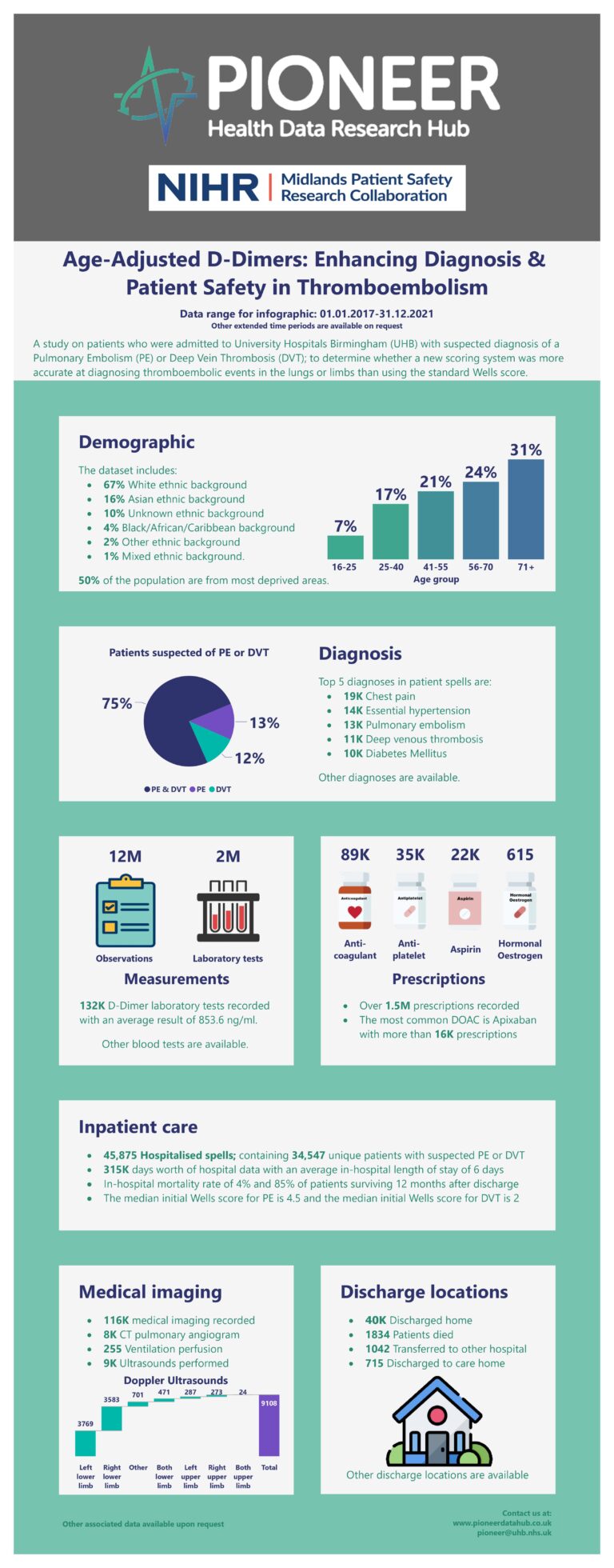
Age-Adjusted D-Dimers: Enhancing Diagnosis & Patient Safety in Thromboembolism
A PIONEER, NIHR Midlands Patient Safety Research Collaborative and NIHR Applied Research Collaboration West Midlands dataset of 27,526 patients with suspected/confirmed thromboembolic events including demographics, physiology, test results and outcomes.
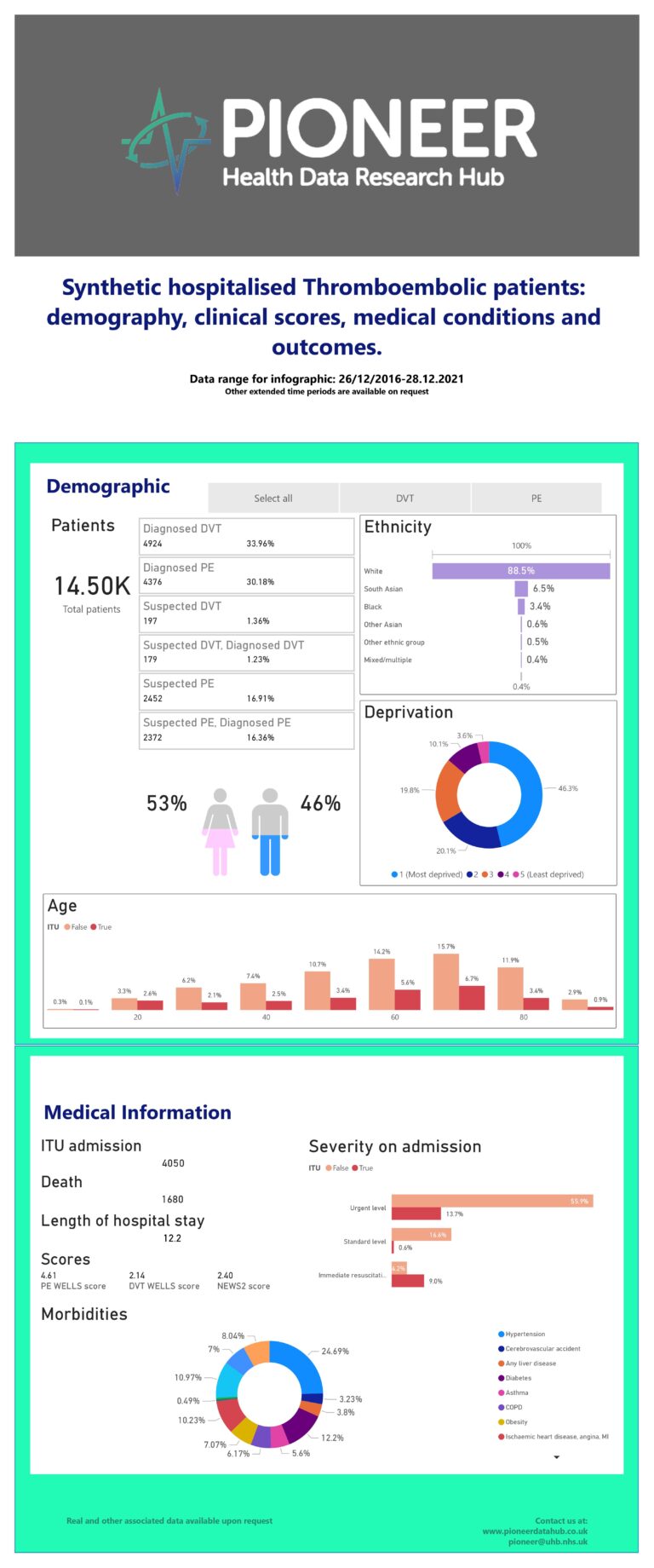
Synthetic Dataset: Hospitalised Patients with Thromboembolic Diagnosis
The incidence of blood clots in the lungs (PE) or limbs (DVT) is estimated to be approximately 50–150 per 100,000 people and in the UK, around 60,000 cases of PE and 200,000 cases of DVT are reported each year. However, despite the significant progress, diagnosing PE and DVT remains a challenge. This large synthetic data with up to 14.5k patients of both suspected and diagnosed thromboembolic events provides key parameters to support critical research into the condition.
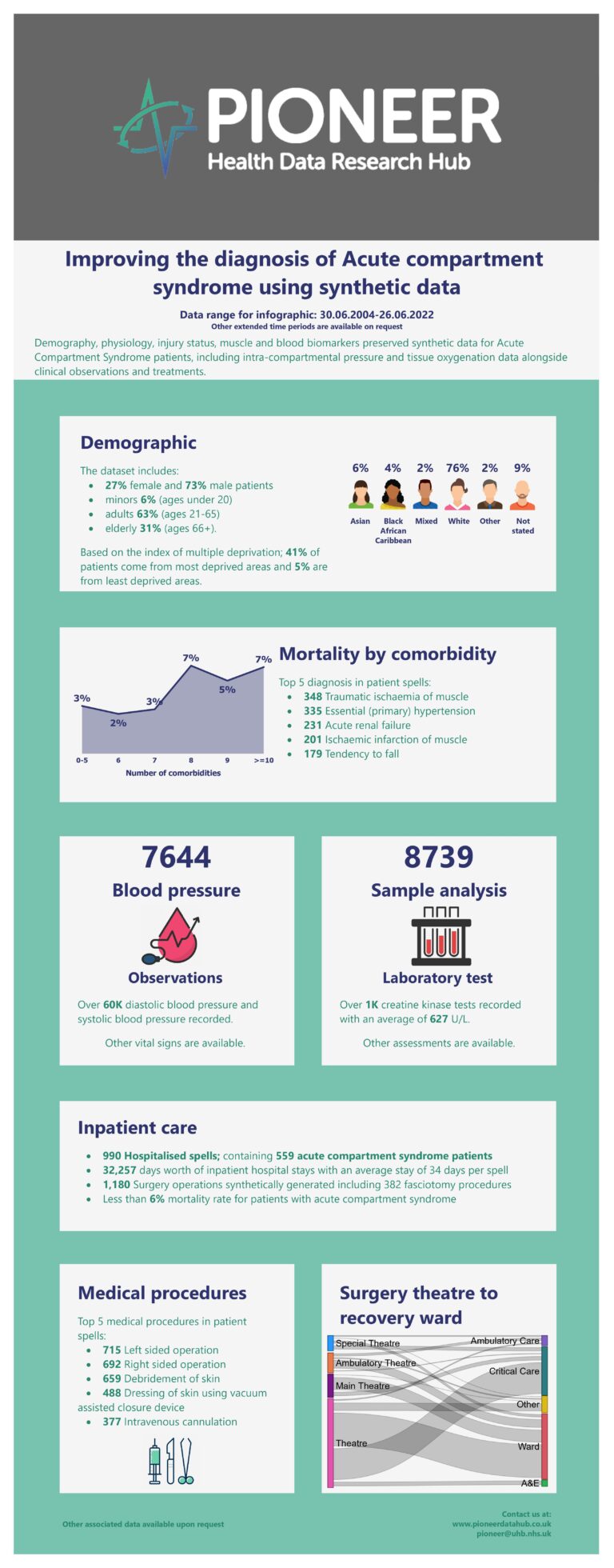
Synthetic Dataset: Using Data-driven ML Towards Improving Diagnosis of ACS
Acute compartment syndrome (ACS) is an emergency orthopaedic condition wherein a rapid rise in compartmental pressure compromises blood perfusion to the tissues leading to ischaemia and muscle necrosis. In this dataset, highly granular synthetic data of over 900 patients with ACS is shown to provide the key parameters to support critical research into this condition.
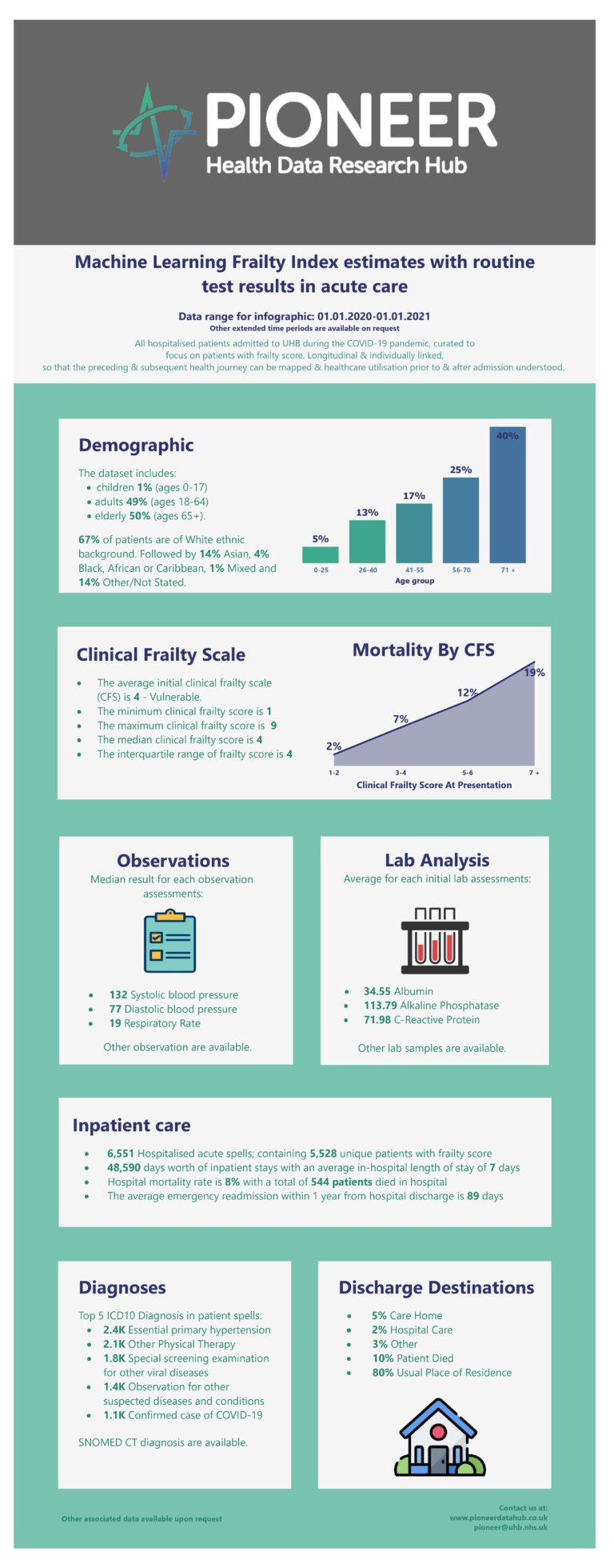
Machine Learning Frailty Index Estimates with Routine Test Results in Acute Care
Frailty is a critical measure in health care for evidence-based clinical decision making. An accurate electronic Frailty Index (eFI) at admission will be beneficial to both patients and medical service for prompt and appropriate assessment and management in acute care. An eFI that was derived from 31 routinely collected test results showed that it has promising identification power for high-risk frailty patients in aged cohort (>65), indicating the potential of a simpler and more efficient model for frailty estimation.
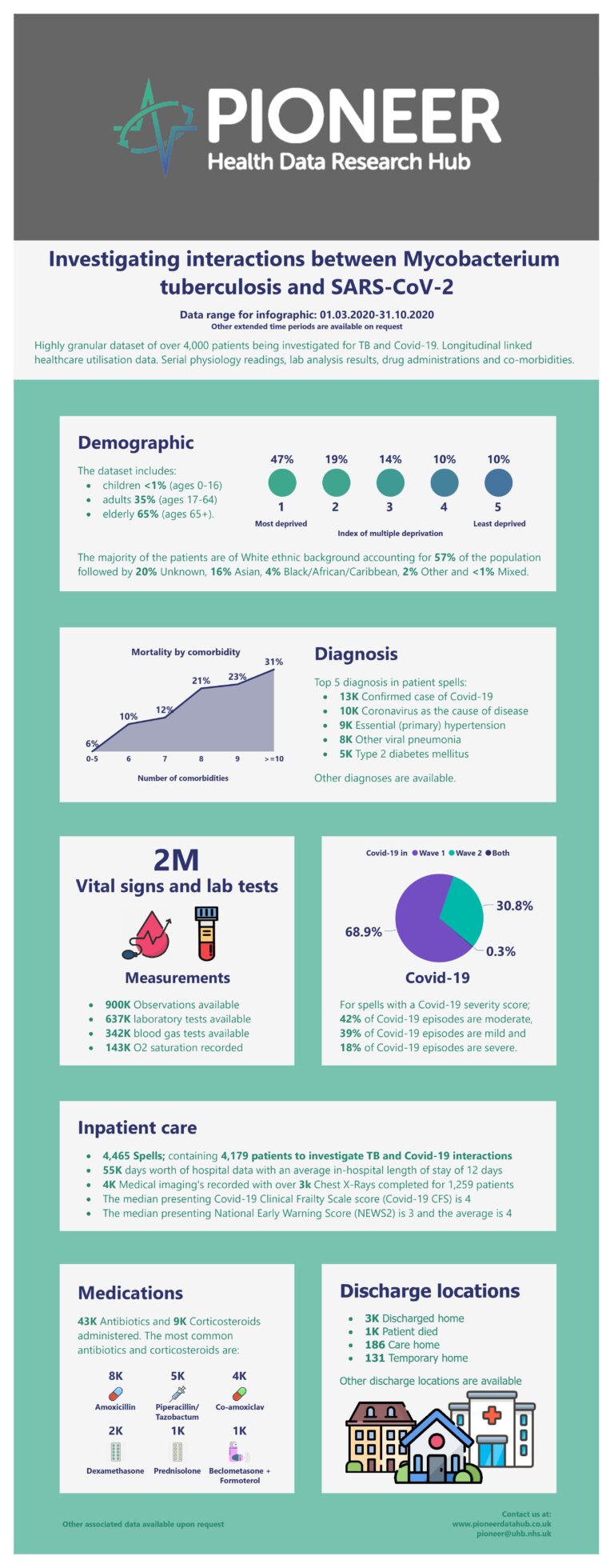
Investigating Interactions Between Mycobacterium Tuberculosis and SARS-CoV-2
Tuberculosis (TB) remains a significant global health problem. The UK has one of the highest rates of TB in Europe, and Birmingham and the West Midlands are hotspots, with over 300 cases of active disease and approximately 10 times that of new latent infections diagnosed each year.
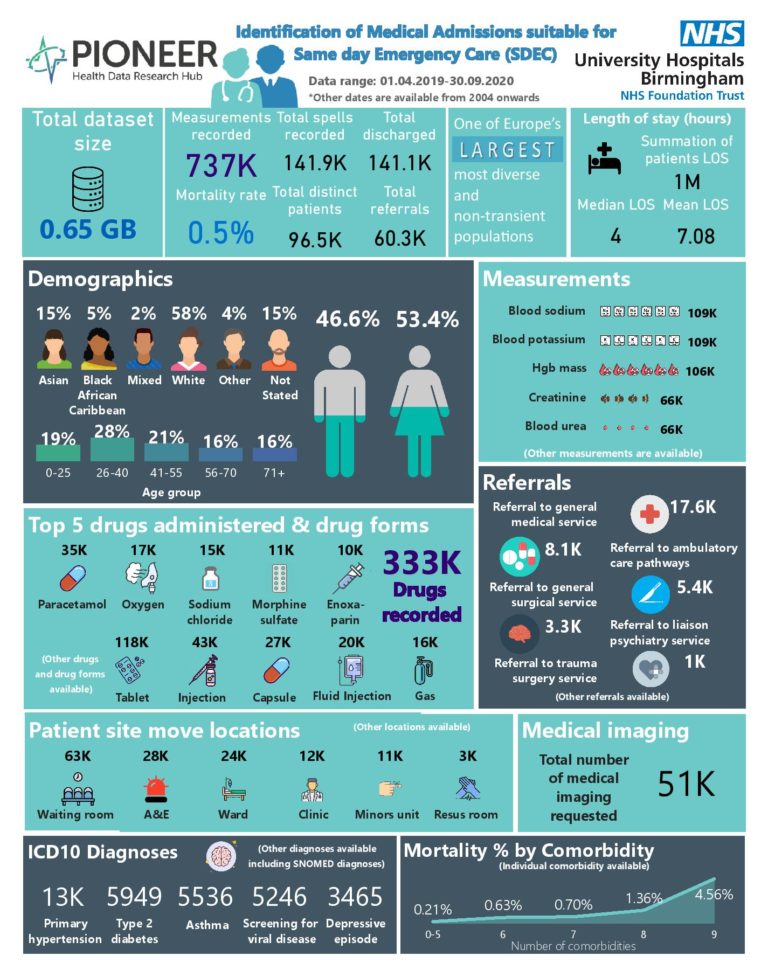
Identification of Medical Admissions Suitable for Same Day Emergency Care
Same Day Emergency Care (SDEC) is beneficial for patients, as hospital admission and its associated risks can be avoided. This dataset includes all acute medical admissions to University Hospitals Birmingham NHS Trust (UHB) from January 2004 to September 2020 onwards.
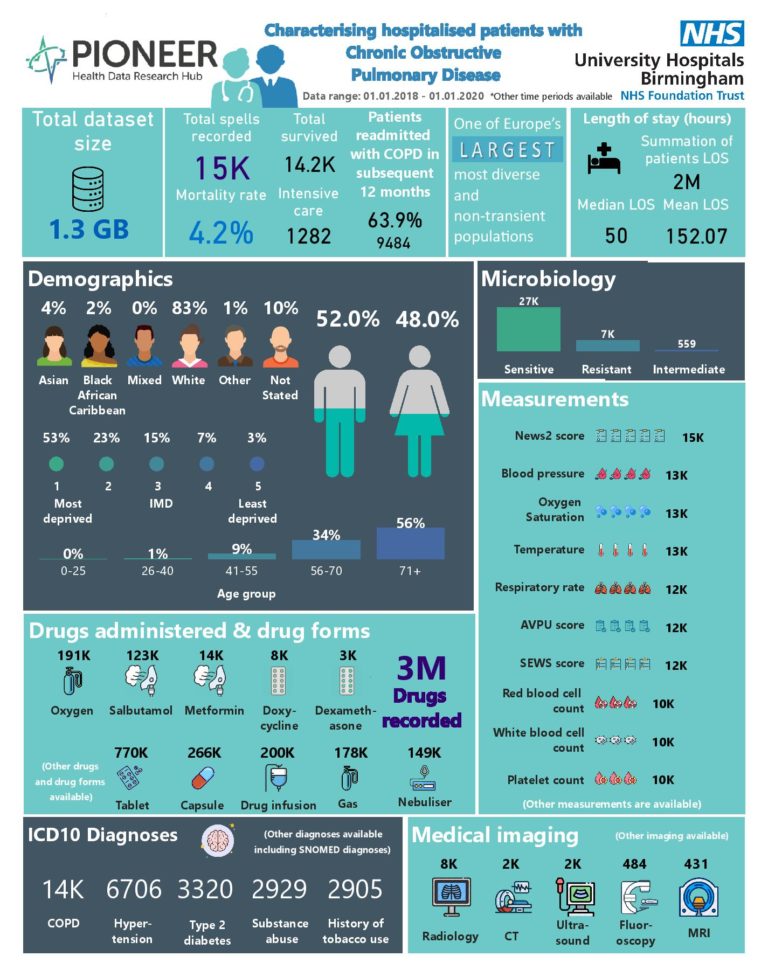
Characterisation of hospitalised COPD exacerbations using real world data
Chronic respiratory diseases remain one of the leading causes of death from non-communicable disease, with the majority of deaths due to Chronic Obstructive Pulmonary Disease (COPD). COPD presents a significant healthcare burden and is detrimental to quality of life. Currently, there are no disease modifying treatments.
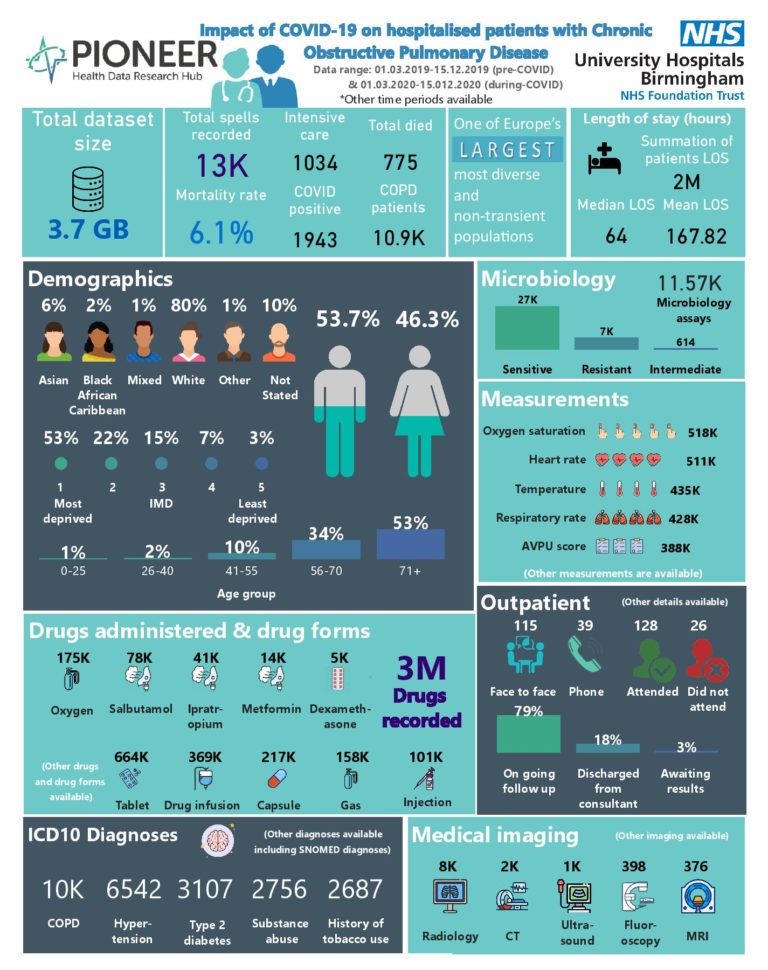
The impact of COVID on hospitalised patients with COPD and hospital services
This dataset explores the impact of hospitalisation and service use in patients with COPD during the COVID pandemic.
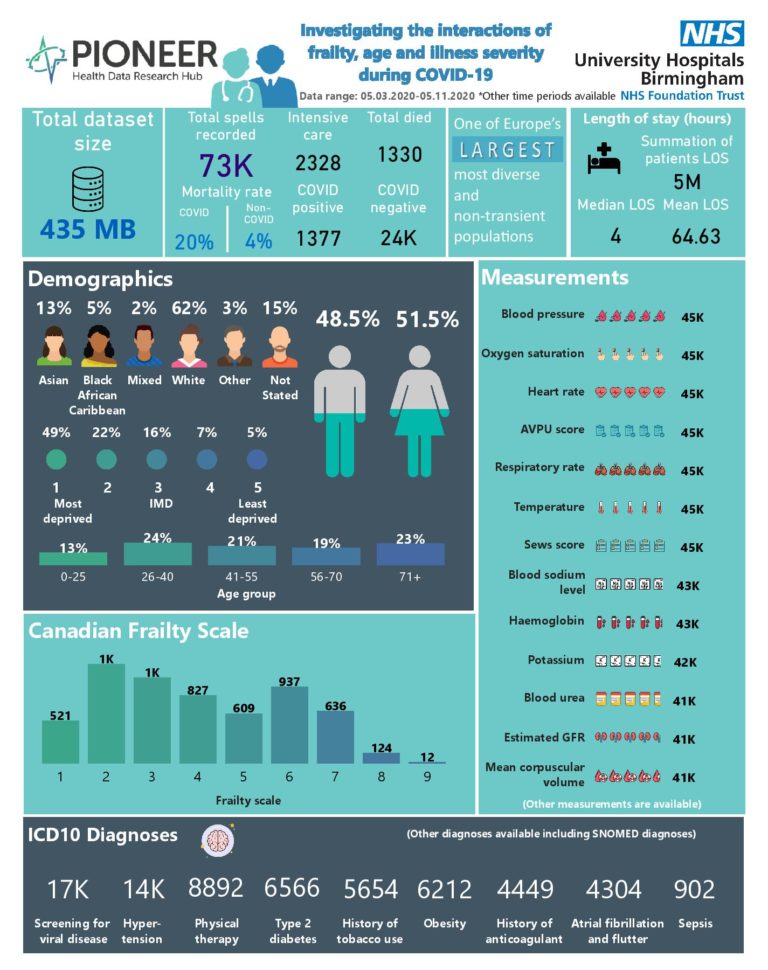
Investigating the impact of frailty, age and illness severity during COVID-19
Frailty is a syndrome of increased vulnerability to incomplete resolution of homeostasis following a stressor event and it is associated with poor outcomes including increased mortality and reduced quality of life. Prevalence increases with age, but it should not be considered an inevitable consequence of ageing.
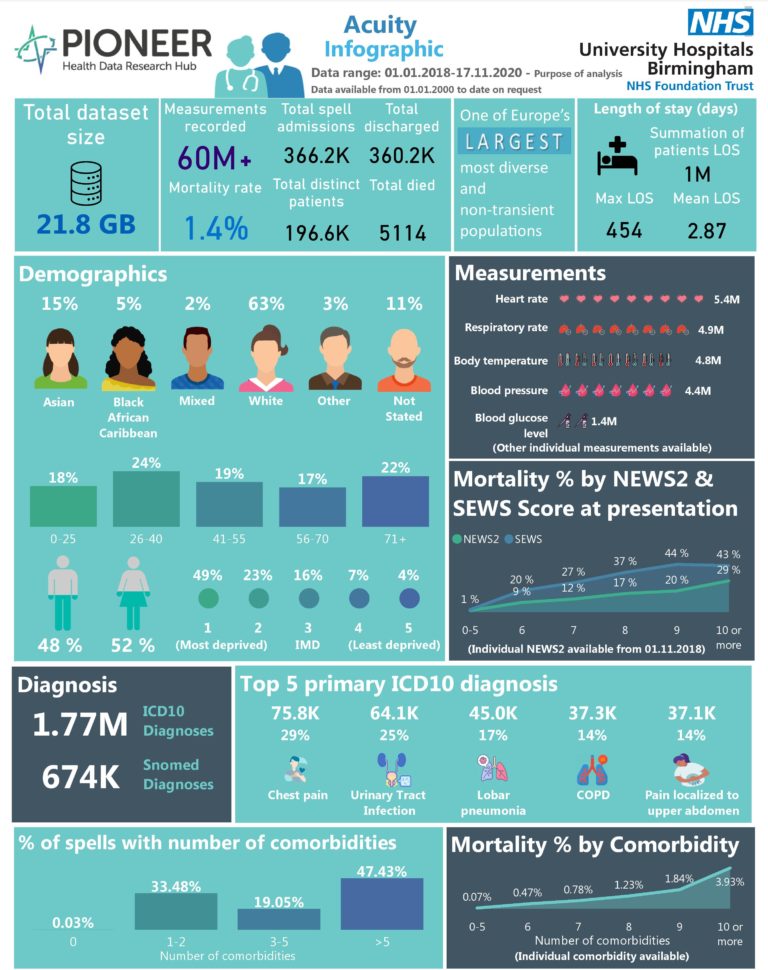
Clinical response thresholds (acuity) in acutely unwell patients: onset-outcome
Early warning systems (EWS) are bedside tools used to assess basic physiological parameters to identify patients with potential or established critical illness.
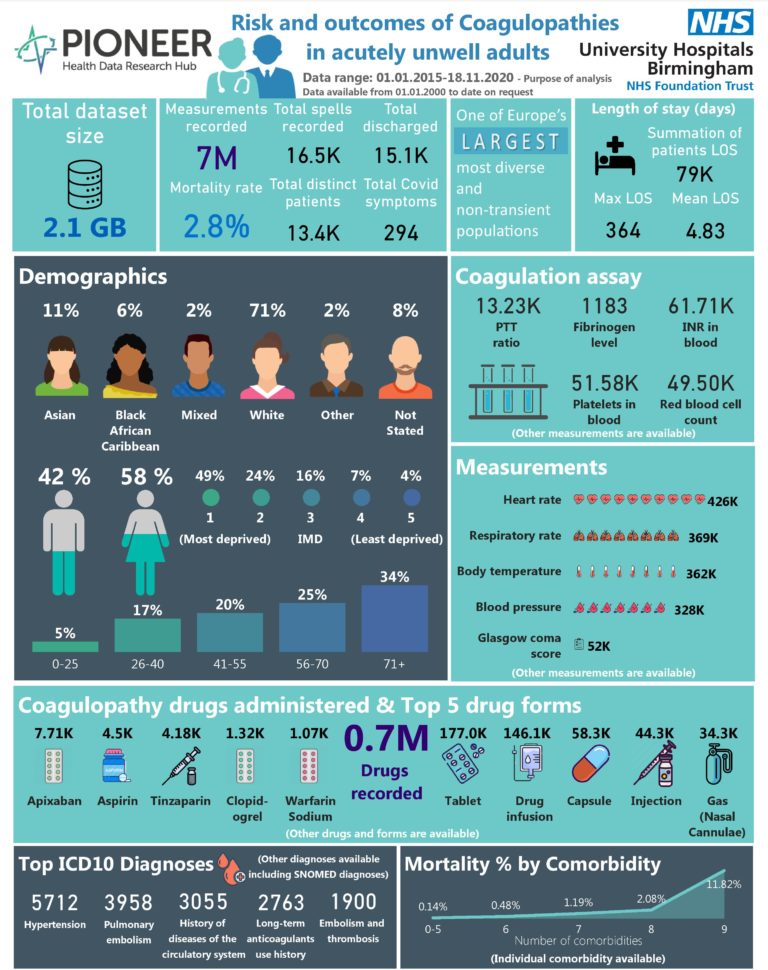
Risk and outcomes of coagulopathies in acutely unwell adults
Coagulopathies and bleeding disorders can reflect hereditary conditions such as Haemophilia or von Willebrand disease, be associated with other diseases such as liver conditions, sepsis, trauma or be iatrogenic, related to therapies or their side effects.
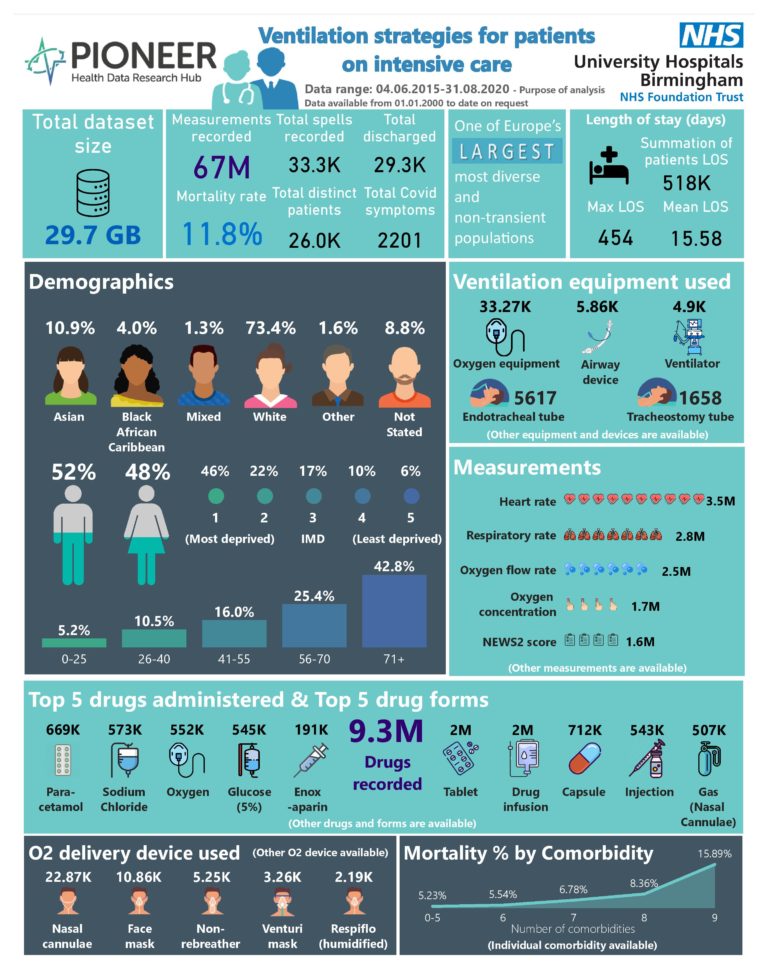
Ventilation strategies for patients on intensive care
Acute respiratory failure is commonly encountered in the emergency department (ED). Early treatment can have positive effects on long-term outcome.
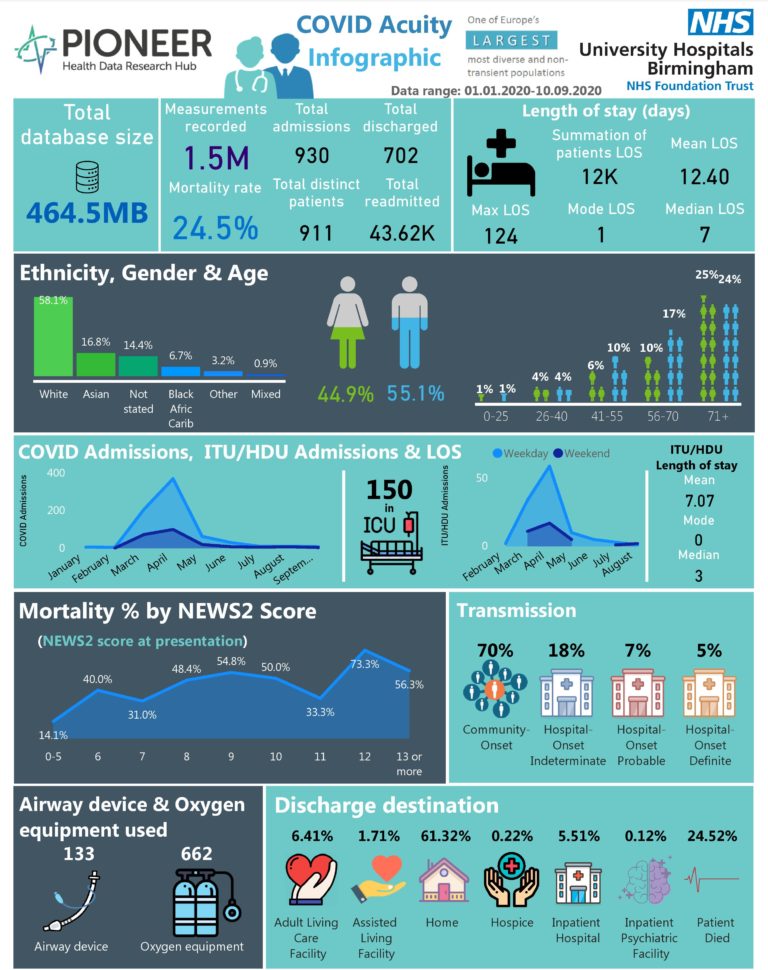
Deeply-phenotyped hospital COVID patients: Acuity, severity, therapies, outcomes
Acuity scores are composite scores which help identify patients who are more unwell to support and prioritise clinical care.
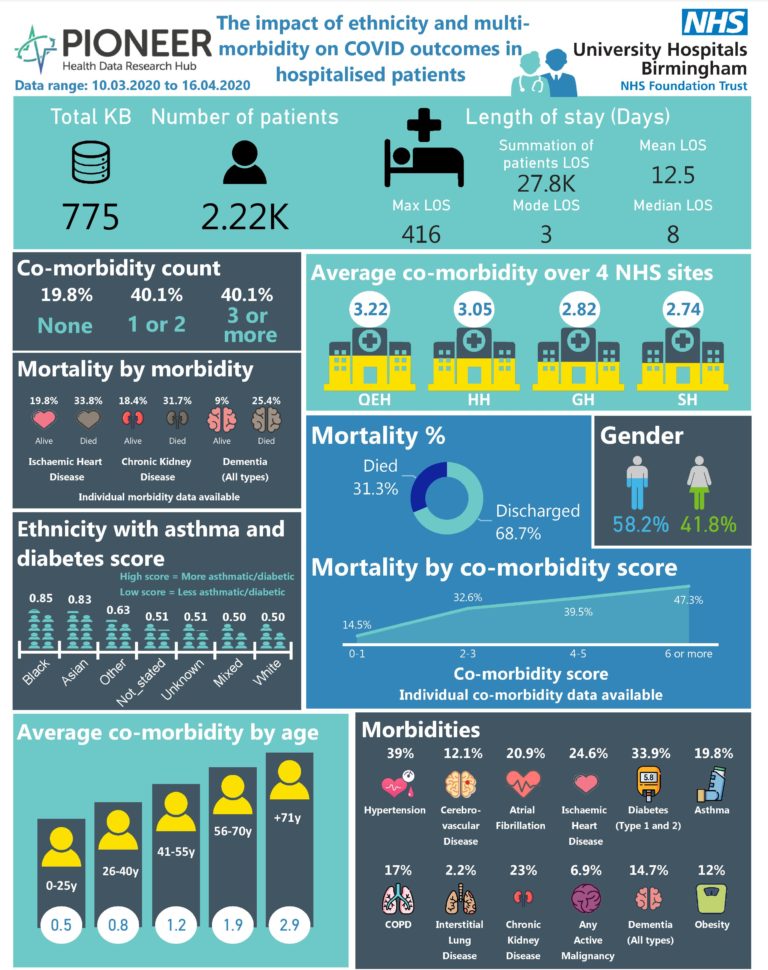
The impact of ethnicity and multi-morbidity on C19 hospitalised outcomes
Some individuals experience severe manifestations of infection, including adult respiratory distress syndrome (ARDS) and death.
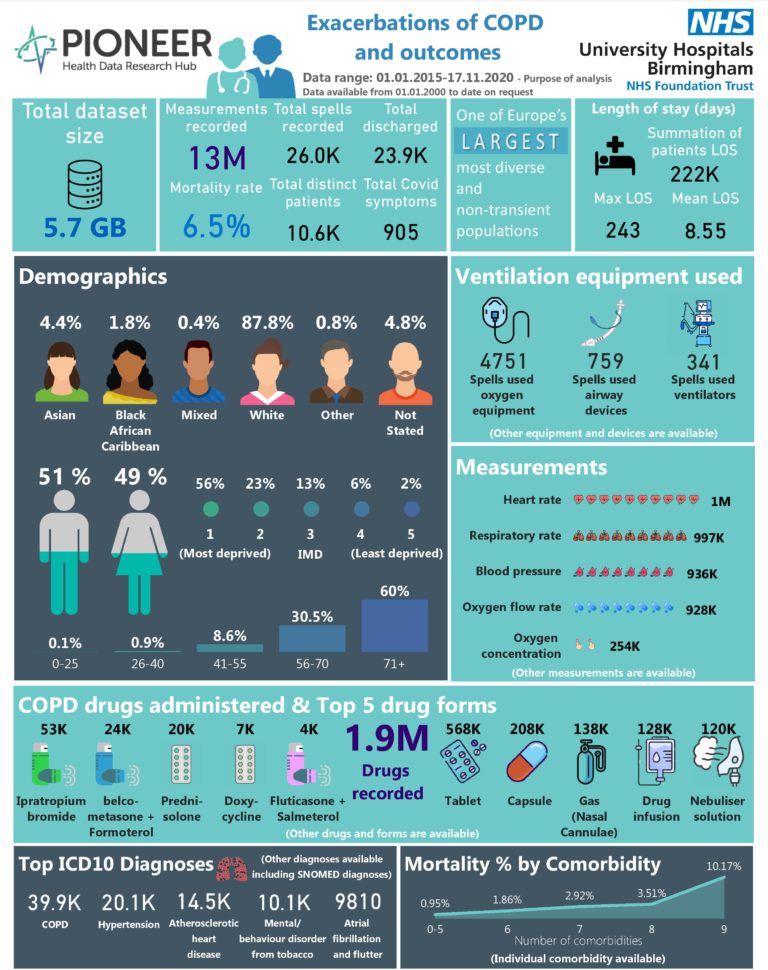
The impact of hospitalised patients with COPD: from admission to outcome
Chronic obstructive pulmonary disease (COPD) is a debilitating lung condition characterised by progressive lung function limitation.
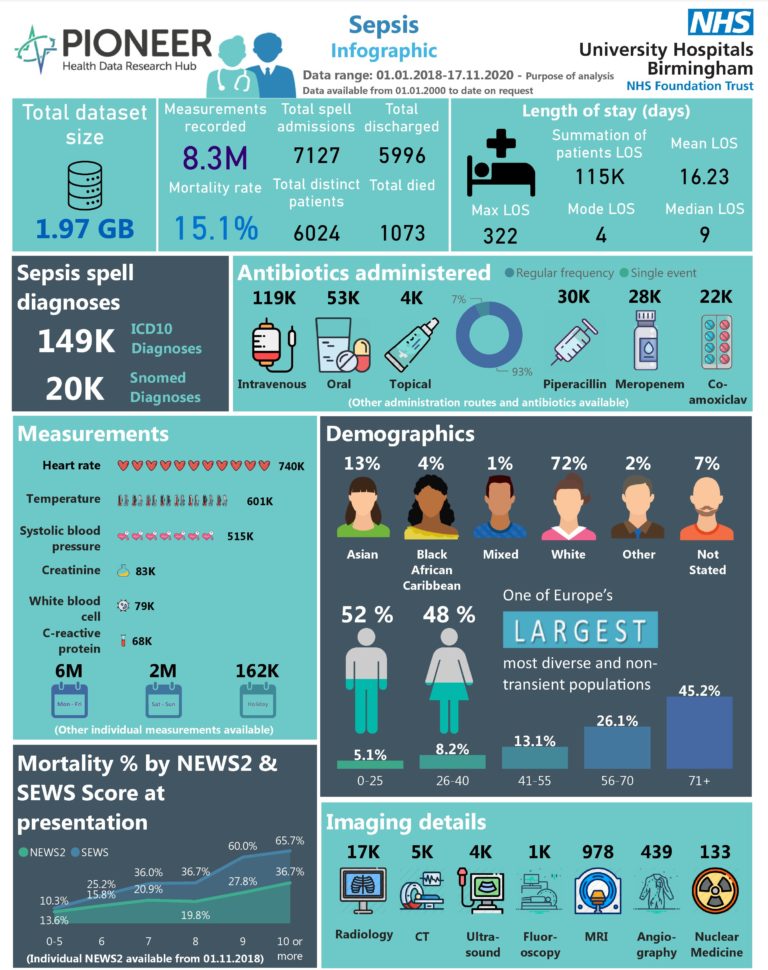
Deeply phenotyped sepsis patients within hospital: onset, treatments & outcomes
Sepsis is life-threatening organ dysfunction due to a dysregulated host response to infection and is a global health challenge.
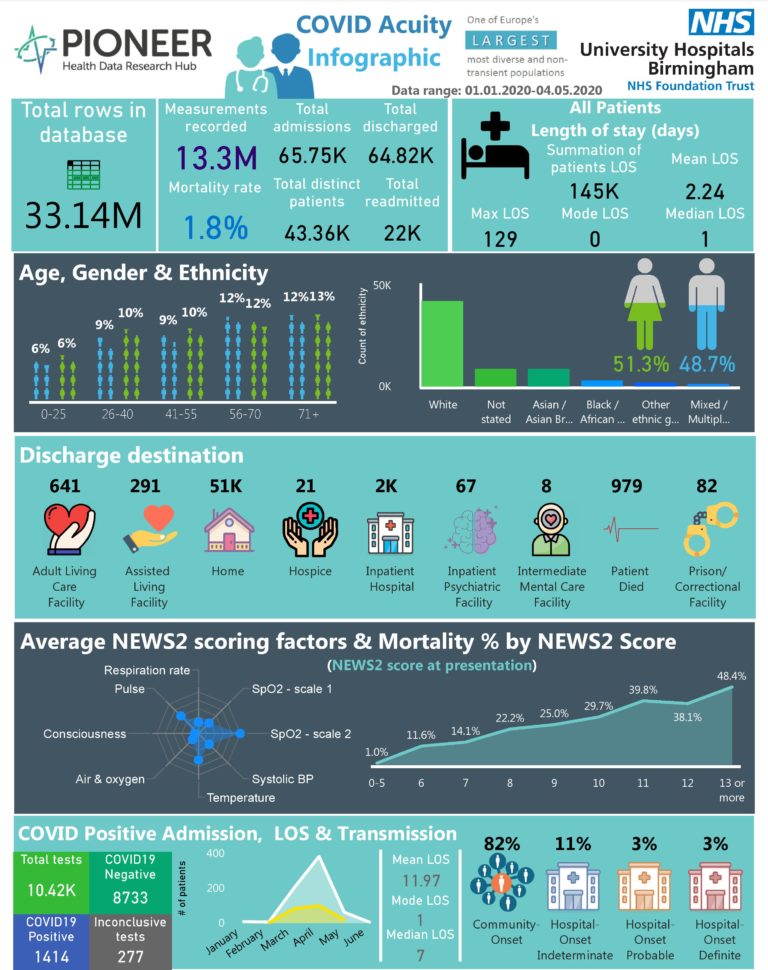
OMOP dataset: Hospital COVID patients: severity, acuity, therapies, outcomes
Coronavirus disease 2019 (COVID-19) was identified in January 2020. Currently, there have been more than 6 million cases& more than 1.5 million deaths worldwide.
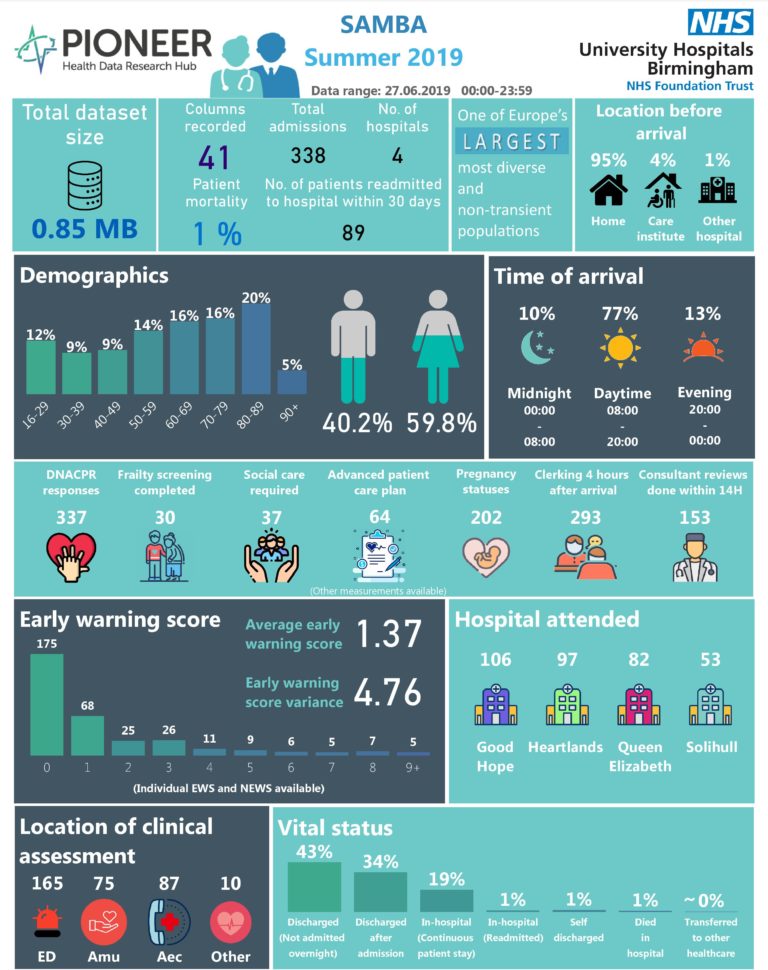
2019 Summer Society of Acute Medicine Benchmarking Audit Hospital care pathways
The Society for Acute Medicine (SAM) Benchmark Audit (SAMBA) is a national benchmark audit of acute medical care.
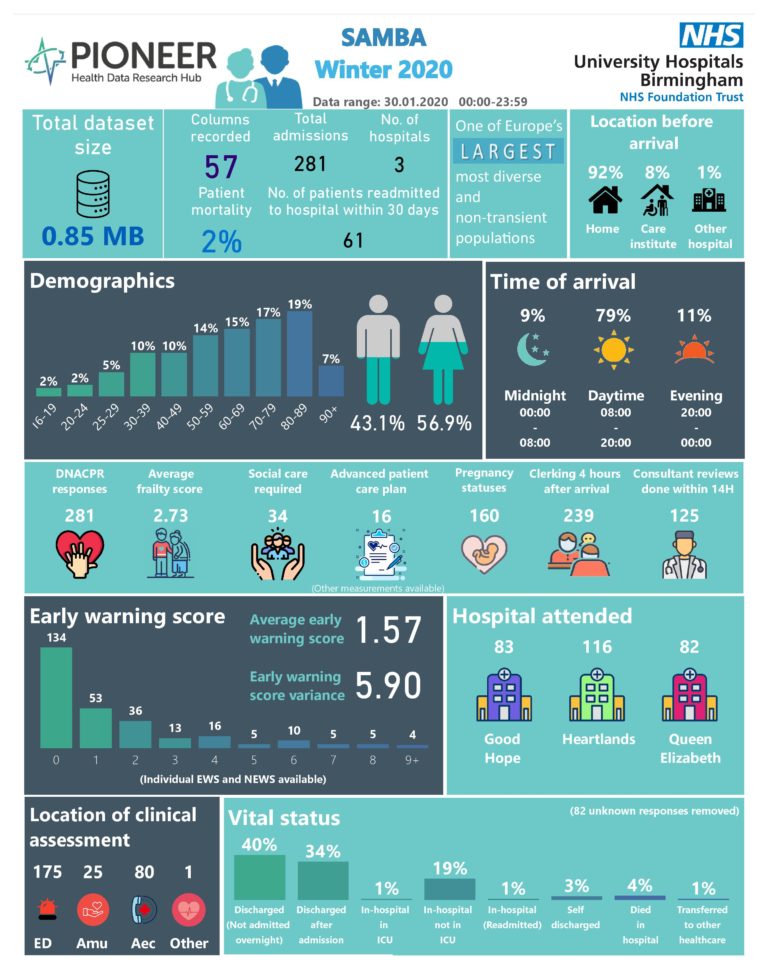
Winter 2020 Society of Acute Medicine Benchmarking Audit Hospital care pathways
The Society for Acute Medicine (SAM) Benchmark Audit (SAMBA) is a national benchmark audit of acute medical care.
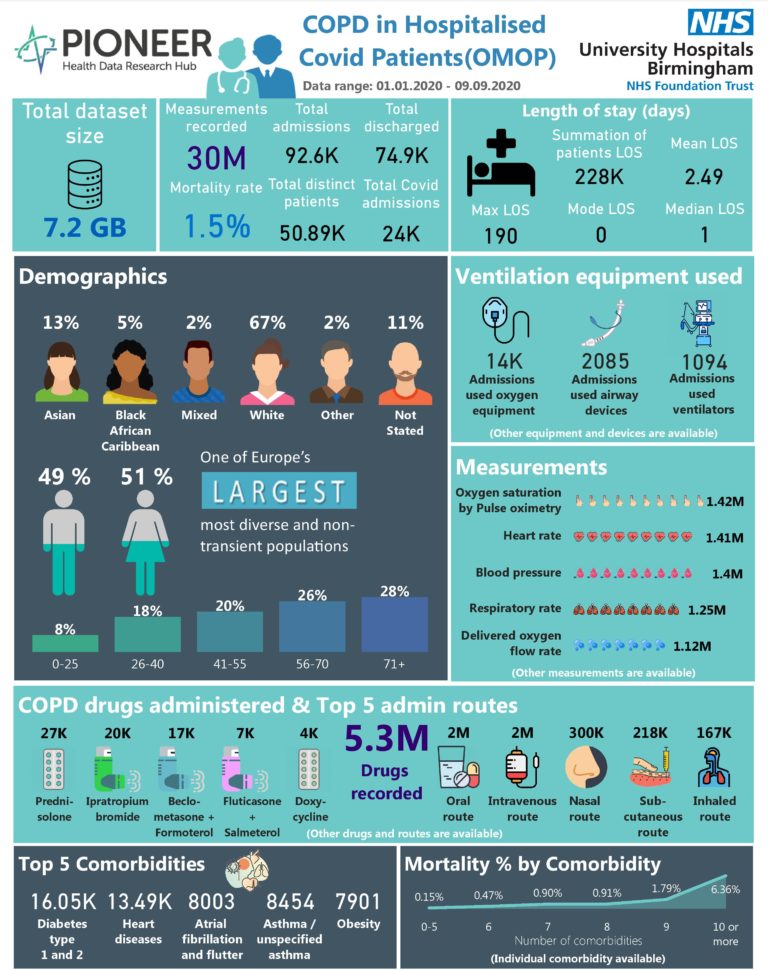
The impact of COVID on hospitalised patients with COPD: a dataset in OMOP
Chronic obstructive pulmonary disease (COPD) is a debilitating lung condition characterised by progressive lung function limitation.
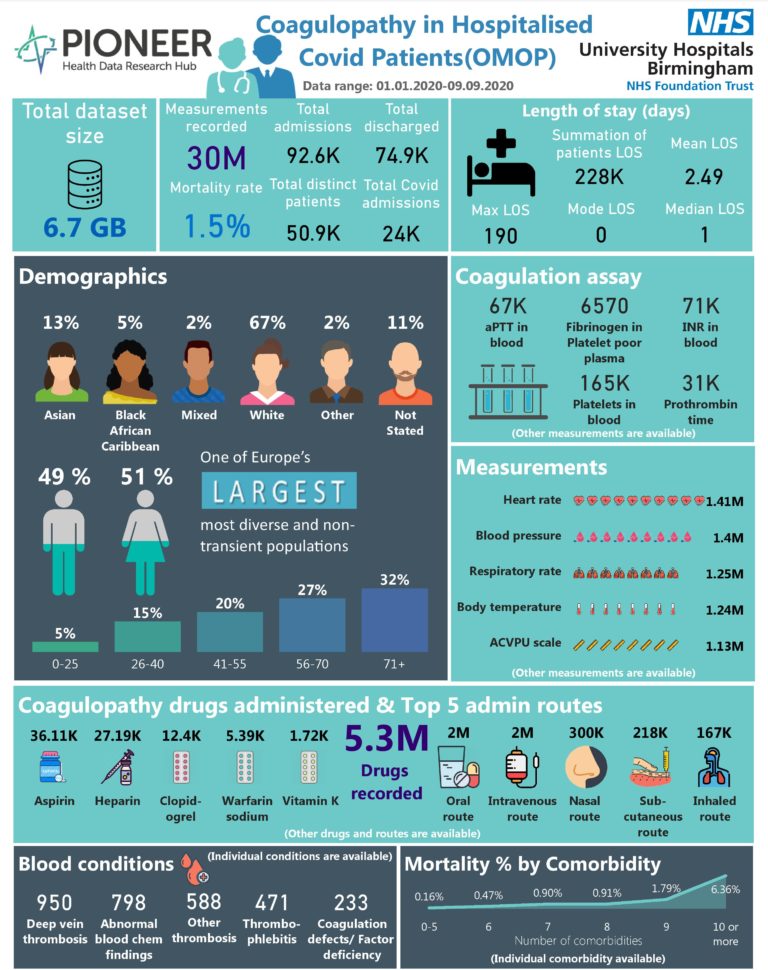
Coagulopathies & arterial/venous thrombosis in COVID patients: an OMOP dataset
In December 2019, the first case of severe acute respiratory syndrome coronavirus 2 (SARS-COV-2) was described and by March 2020, the World Health Organization had declared the disease a pandemic.
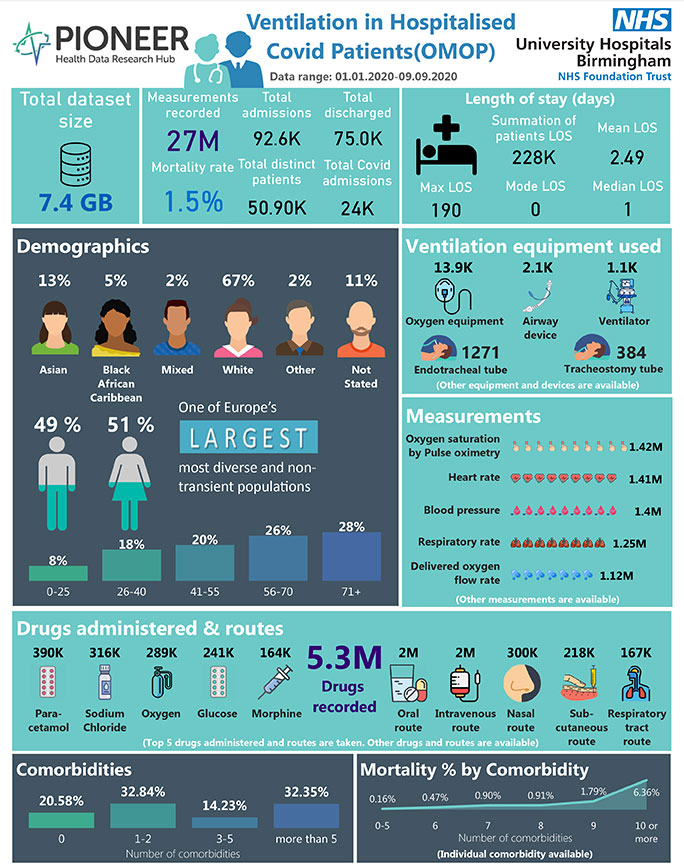
Ventilatory strategies and outcomes for patients with COVID: a dataset in OMOP
Coronavirus disease 2019 (COVID-19) was identified in January 2020. Currently, there have been more than 6 million cases & more than 1.5 million deaths worldwide.